As recently discussed on The Carbon Copy with Brian Janous, utilities are seeing major forecasted demand growth for the first time in decades, and almost entirely from data centers. These data centers are running the internet, serving videos, hosting blogs etc, but most of their projected growth comes from new AI applications, in particular through the major Silicon Valley tech companies (Microsoft, Google, Apple, Facebook, etc) all of whom are looking to put gigawatts of additional data center demand on the grid.
These data centers, as concentrated loads, face much the same problem as new renewable development does being concentrated sources: inadequate grid capacity. While Janous’ new company aims to improve grid utilization through upgraded sensors, there is only so far that upgrades of the existing grid can go. Sooner or later, we’re going to have to break new ground to feed the beast.
The challenge with grid development is that it’s a permitting and construction nightmare, and like many things in the West, has gotten extremely expensive and time consuming to execute. But the datacenters need power much sooner than conventional development can build new power plants and transmission lines – even if permitting, eminent domain, technology, and construction costs were a solved problem!
It is time to lift the constraint that a new data center must be attached to the grid. Instead, we can provide most of the power locally using “beyond the grid” renewable energy generation, backed up by batteries for energy storage overnight.
It is fortuitous that AI data center demand is developing in the mid 2020s, since solar and batteries have been on a 40 year cost progress curve and are now arriving at the point where this is actually possible. In a previous post I talked about application-specific solar for synthetic fuels and for sea water desalination. Here, I unpack the economics of powering AIs with new build solar.
First, a quick refresher on solar and battery cost progress. I recently prepared these two graphs that show the learning rate for solar and batteries. The learning rate, defined as the cost decrease per doubling of production, applies to modular manufactured goods built in a factory, where process controls and innovation can result in continual improvement. For solar, this is currently running at about 44%, while for batteries it’s about 23%, reflecting their relative complexity and immaturity of the manufacturing process. That said, battery production is expanding somewhat faster than solar, so both processes are seeing year-over-year cost improvements in the mid-to-high teens of percents. As of 2024, of course – both technologies are increasing their TAM at a terrifying rate and will probably continue to accelerate.
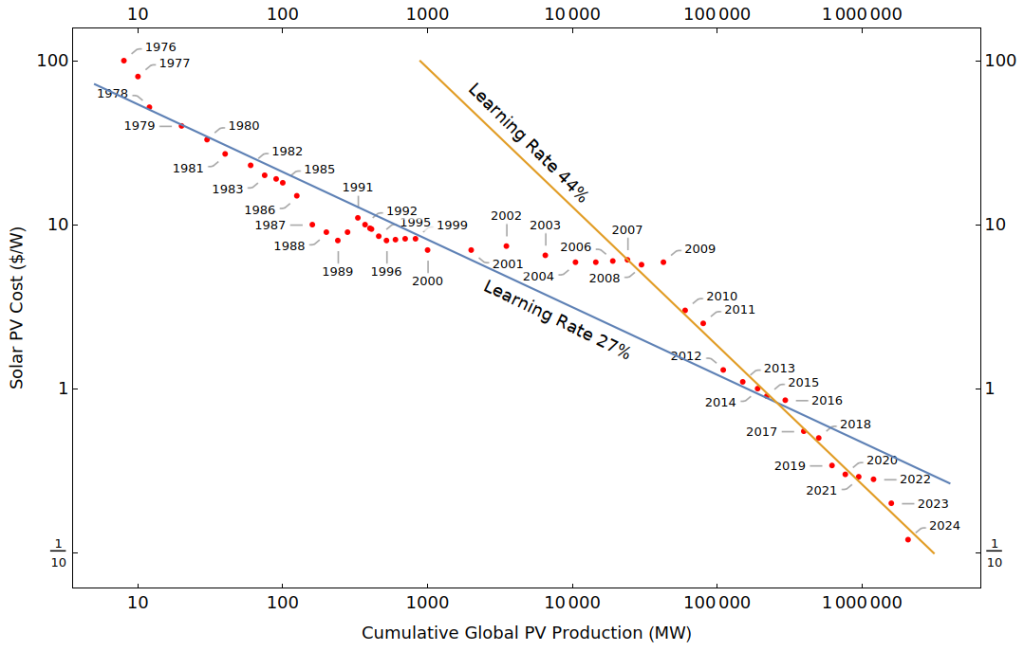

Synthetic fuels, being a low cost commodity, must minimize capex devoted to energy storage and run off pure solar during the day. Data centers, on the other hand, have relatively high fixed costs (somewhere around $50/W) and so profitable operation depends on achieving high utilization.
I recently computed the minimum cost mix for any desired utilization, and showed how these development costs will evolve over the coming decade with future declines in solar and battery costs. Note that even with today’s relatively high solar and battery costs, running a 24/7 operation requires <$4/W capex for power supply, less than 10% of the data center cost.

Of course, there will be times that poor weather reduces battery charging, and by extension, data center up time. This can be addressed by adding additional solar and batteries until profit declines. For example, a data center built in 2028 could have a doubly redundant solar+battery power source for about $4/W, which is very affordable. The trend is clear – we can get to >99.9% data center utilization with relatively affordable overbuild of solar and batteries.
What is this going to look like at scale?
A 1 GW data center (containing roughly a million H100s!) would have a substantial footprint of 20,000 acres, almost all of that solar panels. The batteries for storage and data center itself would occupy only a few of those acres. This is in some sense analogous to a relatively compact city surrounded by extensive farmland to produce its food.
Of course future data centers will use more advanced and productive computers but their power consumption and heat generation will remain much the same. Radically more efficient panels, batteries, and computers will only increase the revenue per unit land used for this purpose.
The development cost of a 1 GW data center would be around $60b including the solar+battery power plant, and importantly the lead time and permitting complexity for each of the components: solar array, batteries, structures, racks, internet connection, and servers is about the same. This is in direct contrast to conventional on-grid power supply, whose capacity is nearly exhausted already.
Is this going to pave the Earth with solar?
My startup Terraform Industries looks to apply solar to produce synthetic fuel, consuming substantial amounts of land (though less than agriculture) in the process. Something like 2 billion acres, or 7% of Earth’s land surface area, would be sufficient to provide every man, woman, and child on Earth with US levels of oil and gas abundance and commensurate prosperity. It’s possible to imagine a future where people consume even more than that – widespread personal supersonic transport, for example – but ongoing conversion of land use away from intensive industrial agriculture toward inherently more productive solar synthetics is a clear net win for the environment.
Is this the case for solar AGI? It’s currently hard to imagine Nvidia and friends producing 100 billion or more H100s, but it’s also hard to imagine our collective demand for artificial intelligence will saturate. If we’re spending $60b on a 20,000 acre solar powered data center development, that’s about $3m/acre. Even if the land acquisition budget is only 3% of the budget at $100,000/acre, this is still substantially higher than essentially all land outside of major cities, including prime agricultural land. Is AI a solar panel maximizer, rather than a paperclip maximizer?
Will AIs prefer to live in space instead of on the Earth?
This is a tough question to answer. Today, the major external demands of data centers – power and water – are much easier to get on Earth than in space. Thousands of times easier, at least. One could imagine a fleet of AGI satellites in sun synchronous orbit. If they were based on the Starlink satellite bus, then the GPU substrate would cost about $10/W, plus launch costs of another $30/W or so – as approximate guesses. Substantial progress on Starship could lower this substantially, but it’s hard to imagine space being cheaper to access and operate within than spare land on Earth.
How much compute will we get?
Earth receives about 173,000 TW of power from the sun. Assuming 100% conversion efficiency, 100% coverage and application via Nvidia H100s (60 teraFLOPS each), we can run 247 trillion GPUs for a total of 1.5 x 10^28 FLOPS. Equating FLOPS to synaptic operations, a human brain can perform about 100 petaFLOPS – equivalent to 1000 H100s but consuming just 20 W vs 700 kW for the GPUs! With current GPUs, the global solar datacenter’s compute is equivalent to ~150 billion humans, though if our computers can eventually match neural efficiency, we could support more like 5 quadrillion AI souls.
It seems that AGI will create an irresistibly strong economic forcing function to pave the entire world with solar panels – including the oceans. We should probably think about how we want this to play out. At current rates of progress, we have about 20 years before paving is complete.
When you mentioned that your data center was going to be ~1GW, my ears perked up. What a great example to compare solar/batteries to nuclear power! If I remember correctly, you live in the LA area, so part of your power comes from the same source as mine–the Palo Verde Nuclear Generating Station outside of Phoenix.
I’ll use your data and compare it to data from Wikipedia for Palo Verde.
Solar/Nuclear
capex/W <$4/$3.4 (2022 $)
uptime >99.9%/93% (~6 wk maint every 18 mo, otherwise ~100%)
footprint 20000ac/4000ac (for ~4000 MW capacity)
Seems competitive to me! The only problem I see is with the land use–6×6 mi or 9×9 km and the fact that someone may want to have their data center where there isn’t enough sun (towards the poles or cloudy). I also don’t see any information about the seasonality of solar. For example, one of the sunniest cities in the US, Yuma, has nearly 3x sun in the summer, 3.3 vs 8.6 kWh/m2/d. Is your $4/W based on December? Will Wall Street want to have its own data center (duh)? New York is 1.8 in December, so >$7/W? London 0.7/$19?
LikeLike
This is untrue as a cursory glance at the wikipedia page shows you: https://en.wikipedia.org/wiki/Palo_Verde_Nuclear_Generating_Station#Electricity_Production
The numbers jump around every month, it’s not constant for 18 months then down. And no, it’s not market demand, they are first in line to sell. They also pull the sneaky trick of comparing the thermal generation figures to the nominal electric capacity which is why sometimes the numbers are above 100% nominal output, it helps makes the numbers look better then they are.
That is the overnight construction costs for Palo Verde. The distinction matters enormously when work begins 10 years before the project is finished. That’s 10 years where your power needs to be coming from somewhere else, somewhere you are paying for and most likely something that is polluting.
And unlike solar, nuclear has substantial costs past the initial capital expenditure. They tend to lowball these costs because their business model relies on governments support but the blunt truth is that if their claims about operating costs under 4 cents per kilowatt-hour were remotely true, they would not be needing subsidies for their operating costs and shutting down if the government balks at those subsidies.
LikeLike
I’m not sure if you are not understanding or you are purposely strawmanning what I said. Uptime is not the same as capacity factor: 5.5wk maintenance/18 month running is 93%; the reactors truly work 100% of the time otherwise (if not you would hear about it!). Like other thermal power plants, they can be run anywhere from 50-100% of capacity to match demand, so the numbers “jump around”. There are 3 reactors at Palo Verde, so they have staggered ~18/24 month (and not exactly that!) maintenance schedules, with that happening in the spring or fall where demand is the lowest, which would not allow the reported numbers to go to zero.
No, I did not include interest in construction cost. Yes, reactors currently take 10 years to build; plan ahead. I’m not sure that they are getting subsidies for operating outside of insurance.
News Flash: Nuclear reactors are very capital-intensive power sources with low operating costs. They will run for up to 80(!) years before needing to be decommissioned, producing close to zero greenhouse gases in that time.
Solar takes a bunch of room (needs space), doesn’t produce much power outside of midday (needs storage) and season/location (needs backup), and need to be totally replaced after ~25 years (needs a landfill).
If you want to make people’s heads explode, explain how solar can be paired with nuclear for 100% uptime (including peaking?), year-round (at least in lower latitudes) power!
LikeLike
Two major comments:
First, a fairly prosaic point: AI will likely mature as a low-bandwidth, high-compute application. So putting AI datacenters in extremely remote areas (aka “deserts”) works pretty well. Even if they only had network access via satellites, they’d probably do OK. It’s also easy to run major terrestrial internet backbone to pretty much anywhere these days. The point is that your connectivity solutions don’t need to be as robust as your power solutions.
Another nice thing about remote areas: Permitting is much, much cheaper. If your remote area is somewhere in the developing world, permitting is almost a non-issue (although security and corruption costs go up).
The more interesting point is that AI compute loads fall into to major buckets:
It’d be very interesting to quantify total compute load between these two classes of activity. If, as I suspect, training is a large chunk of the total compute load, then your battery/storage costs go way, way down, and the application looks a lot more like fuel production. You train stuff while the sun shines, and you don’t worry about it when it doesn’t.
LikeLike