Bringing Deep Learning to the Edge: How Edge Computing is Revolutionizing AI
As technology continues to improve exponentially, deep learning has emerged as a critical tool for enabling machines to make decisions and predictions based on large volumes of data. However, complicated deep learning algorithms can strain conventional cloud computing infrastructures, resulting in poorer processing rates, significant security risks, and expensive bandwidth costs.
Edge computing may change how we think about deep learning. Edge computing can significantly increase the speed and efficiency of deep learning models while lowering the pressure on cloud servers by bringing computing capacity closer to the data source. It’s like having a personal assistant with you at all times, ready to help you with any task without needing to call back to headquarters for instructions.
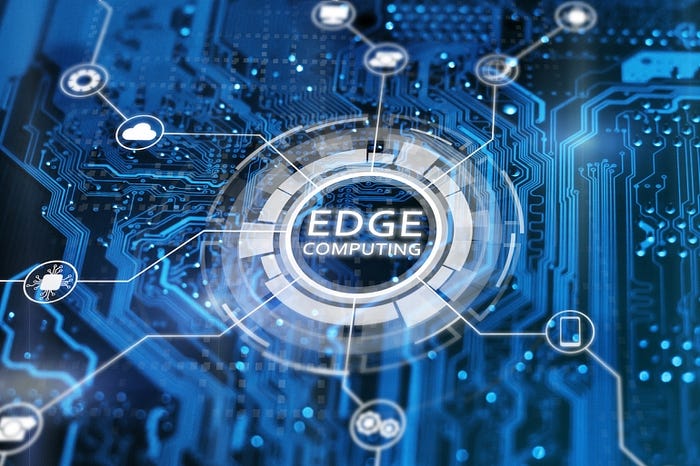
But edge computing isn’t just about convenience. It also offers enhanced privacy and security, lower bandwidth requirements, and greater flexibility and scalability than traditional cloud computing. Edge computing in deep learning is a fascinating field with the potential to revolutionize many different sectors, from healthcare to transportation, and we’ll look at it here.
So come along with us on this journey as we discover the fascinating possibilities of bringing deep learning to the edge!
Standardizing model management can be tricky but there is a solution. Learn more about experiment management from Comet’s own Nikolas Laskaris.
So, What is Edge Computing?
So, what is this thing called “edge computing,” and how does it compare to the more conventional “cloud”? Edge computing is a decentralized computing paradigm that entails processing data close to the point of origin rather than transferring it all to a centralized data centre. This allows sensors, cell phones, and other “edge” devices to process the data they acquire in real-time without maintaining a constant connection to the cloud.
Particularly for deep learning, edge computing has significant benefits over the more conventional cloud. Speed is a major plus. Edge computing can significantly increase the pace of deep learning models, resulting in quicker and more accurate predictions, by shortening the distance between the data and the processing power. Making real-time decisions is crucial for driverless vehicles and medical monitoring equipment applications.
Another advantage of edge computing is enhanced privacy and security. When data is processed locally, it doesn’t have to be sent across the internet or other networks, eliminating one potential source of security issues. In addition, when there is a problem with the network, edge computing can help prevent data loss.
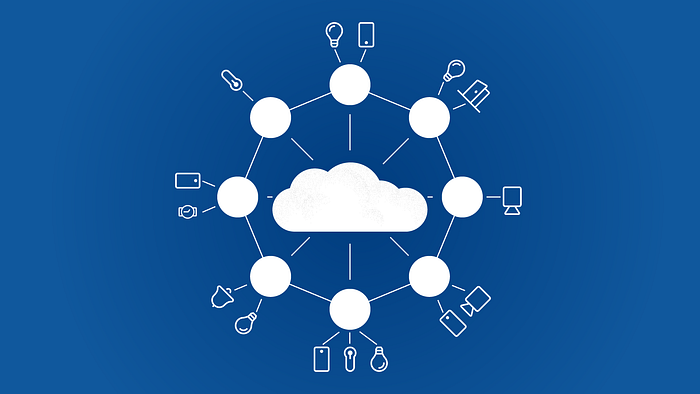
Driving the Future: How Edge Computing is Revolutionizing Deep Learning Applications
Autonomous Vehicles
Autonomous vehicles are one of the most exciting edge computing applications in deep learning. By integrating robust sensors and edge computing capabilities, self-driving cars can make decisions on the fly without relying on a remote server. This allows autonomous vehicles to function in real-time, making split-second judgments to ensure the safety of their passengers and prevent collisions. In addition, edge computing can help reduce autonomous vehicle systems’ latency and bandwidth requirements, leading to more reliable and efficient performance.
From Wearables to Wellness: The Impact of Edge Computing on Healthcare and Monitoring Devices
Another area where edge computing has significant impact is in healthcare and wellness applications. From heart rate to sleep quality and everything in between, wearable gadgets like smartwatches and fitness trackers can record a wealth of information about an individual’s health and wellness. Edge computing allows these devices to process this data in real time, giving users actionable insights into their health and well-being. Additionally, edge computing can help healthcare professionals monitor patients remotely, enabling more efficient and effective care.
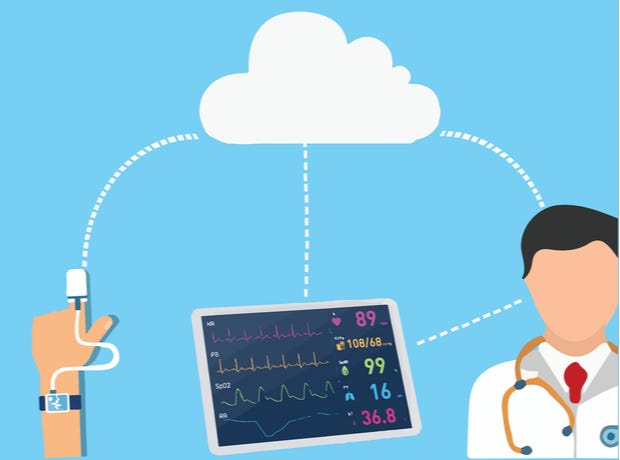
Industrial Internet of Things (IIoT)
Edge computing is also changing how we apply deep learning in the Industrial Internet of Things (IIoT) realm. By integrating sensors and edge computing capabilities into industrial equipment, manufacturers can monitor real-time performance, identify potential issues before they become problems, and optimize efficiency. Additionally, edge computing can help reduce the latency and bandwidth requirements of IIoT systems, making them more reliable and cost-effective.
Challenges and Opportunities of Edge Computing in Deep Learning
Overcoming Latency
One of the biggest challenges of edge computing in deep learning is overcoming latency. While edge computing can reduce the distance between the data and the processing power, there is still a delay between the two. This is a significant issue when quick decisions are required, such as in autonomous vehicles and factories. To overcome this challenge, researchers are exploring ways to optimize edge computing architectures and reduce the latency of deep learning models.
Balancing Security and Privacy
Another challenge of edge computing in deep learning is balancing security and privacy. While edge computing can help reduce the risk of data breaches and other security threats, it raises new concerns about data privacy. Because data is processed locally, there is a greater risk of data exposure if a device is lost or stolen. To address this challenge, researchers are exploring ways to implement more robust encryption and other security measures and developing new privacy-preserving techniques for edge computing.
As Compared With Cloud Computing
Despite its many advantages over conventional cloud computing, edge computing has drawbacks. Some deep learning applications may outgrow the processing resources and storage capabilities at the edge. Researchers are looking for hybrid architectures that combine edge and cloud computing to address this obstacle.
Building Robust Edge Computing Infrastructure
Finally, one of the critical opportunities of edge computing in deep learning is the ability to build a more robust and resilient infrastructure. By distributing computational resources across a network of devices, edge computing can help reduce the risk of system failures and improve the overall reliability of deep learning applications. To fully realize this potential, however, researchers must continue developing new tools and techniques for managing edge computing infrastructure and ensuring it can adapt to changing conditions and demands.
Conclusion: The Future of Edge Computing in Deep Learning
Edge computing is transforming the field of deep learning, enabling new applications and driving innovation across a range of industries. By bringing the processing power closer to the data, edge computing can help reduce latency, increase reliability, and improve efficiency in deep learning applications. However, as we’ve discussed, edge computing also presents new latency, security, and infrastructure challenges.
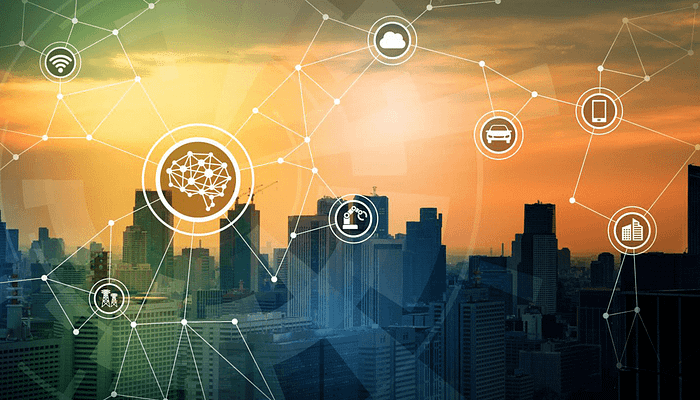
Future Directions
There are several exciting directions for future research in edge computing and deep learning. One area of particular interest is developing more efficient and effective edge computing architectures, which can improve the performance and reliability of deep learning applications. Another area is exploring new techniques for optimizing edge computing infrastructure, such as using machine learning to predict resource demands and automate resource allocation.
Final Thoughts
Deep learning will surely benefit from the further development of edge computing. From autonomous vehicles to healthcare and industrial applications, edge computing pushes the boundaries of what is possible with AI. By leveraging the power of edge and cloud computing, researchers and industry professionals can develop more robust and resilient systems, unlocking new opportunities for innovation and growth.
If you’d like to read more on this, here are some few references that could come in handy:
[1] Cao, K., et all (2020). An overview on edge computing research.
[2] Shahid L., et all (2021)Deep Learning for the Industrial Internet of Things (IIoT)
[3] Stephen J. What is edge computing? Everything you need to know
Editor’s Note: Heartbeat is a contributor-driven online publication and community dedicated to providing premier educational resources for data science, machine learning, and deep learning practitioners. We’re committed to supporting and inspiring developers and engineers from all walks of life.
Editorially independent, Heartbeat is sponsored and published by Comet, an MLOps platform that enables data scientists & ML teams to track, compare, explain, & optimize their experiments. We pay our contributors, and we don’t sell ads.
If you’d like to contribute, head on over to our call for contributors. You can also sign up to receive our weekly newsletter (Deep Learning Weekly), check out the Comet blog, join us on Slack, and follow Comet on Twitter and LinkedIn for resources, events, and much more that will help you build better ML models, faster.